- Research
New Research Validates RM Compare Ranks as Effective On-Demand Rulers
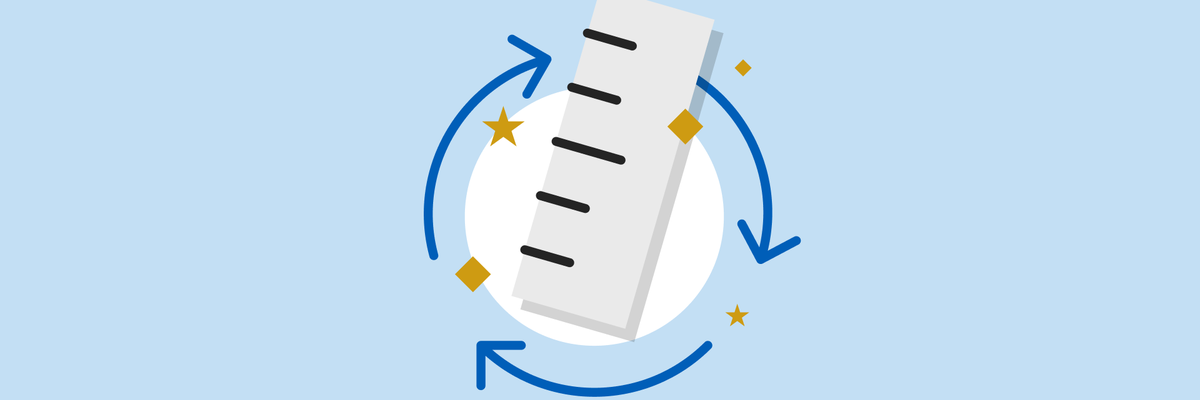
In the ever-evolving landscape of educational assessment, Adaptive Comparative Judgement (ACJ) continues to prove its worth as a powerful and flexible tool. A recent study by Jeffrey Buckley, published in the Assessment in Education: Principles, Policy & Practice journal, provides compelling evidence that further validates the effectiveness of ACJ ranks, particularly those generated by systems like RM Compare, as on-demand rulers for assessment purposes.
Want to learn more? Listen to the deep dive.
Key Findings
Buckley's research, titled "Modelling approaches to combining and comparing independent adaptive comparative judgement ranks," delves into the intricacies of ACJ and offers several important insights:
- Consistency Across Independent Sessions: The study demonstrates that independent ACJ sessions, when properly conducted, produce remarkably consistent rank orders. This finding supports the reliability of ACJ as an assessment method and reinforces the validity of using RM Compare ranks as measurement tools.
- Effective Combination of Ranks: Buckley's research explores various methods for combining ranks from independent ACJ sessions. The results indicate that these combined ranks maintain high levels of consistency, further solidifying the robustness of ACJ-derived measurements.
- Validation of On-Demand Rulers: Perhaps most significantly for RM Compare users, the study provides strong evidence supporting the use of ACJ ranks as on-demand rulers. This means that the rank orders generated through RM Compare can be confidently used as reliable measurement scales for assessing student work or other items
Implications for RM Compare Users
These findings have several important implications for educators, researchers, and assessment professionals using RM Compare:
- Enhanced Confidence: Users can have increased confidence in the reliability and validity of the rank orders produced by RM Compare sessions.
- Flexible Assessment Options: The validation of ranks as on-demand rulers opens up new possibilities for flexible and efficient assessment practices.
- Scalability: The ability to combine ranks from independent sessions suggests that RM Compare can be effectively used for larger-scale assessments without compromising reliability.
- Continuous Improvement: This research provides a solid foundation for further refinement and development of ACJ methodologies within RM Compare.
Looking Ahead
As we continue to develop and enhance RM Compare, research like Buckley's plays a crucial role in guiding our efforts. The validation of ACJ ranks as effective on-demand rulers reinforces the value of our platform in supporting fair, reliable, and efficient assessment practices.
We're excited about the possibilities this research opens up and are committed to incorporating these insights into future updates and features for RM Compare. Stay tuned for more developments as we work to provide you with the most advanced and reliable ACJ tools available.
For those interested in diving deeper into the research, we encourage you to read Buckley's full paper, which offers a wealth of detailed analysis and insights into the world of Adaptive Comparative Judgement.
As always, we welcome your thoughts and feedback on how these findings might impact your use of RM Compare. Let's continue to push the boundaries of what's possible in educational assessment together!
FAQ
FAQ: Adaptive Comparative Judgement (ACJ) for Large-Scale Assessments
What challenges arise when using ACJ for national testing with large cohorts?
Managing a single ACJ session with thousands of participants poses significant challenges. Firstly, it demands a substantial number of judgments from each judge, potentially leading to judge fatigue and incomplete data. For instance, with 6,000 portfolios, each of the 200 judges would need to make 300 judgments. Secondly, handling such a large dataset becomes computationally intensive and logistically complex.
How does the proposed 'Steady State' approach address these challenges?
The 'Steady State' approach proposes conducting ACJ in smaller, localized cohorts, producing individual ranks that are then merged into a national rank. This minimizes the impact of incomplete judgments and simplifies logistics by dealing with smaller, geographically closer groups.
What were the three main aims guiding this research project?
The project had three key aims:
- Aim 1: Determine if merging discrete ACJ ranks is reliable and develop a method to assess the reliability of this process.
- Aim 2: Investigate methods for optimizing the merging process to enhance reliability and closely approximate the results of a single, large-scale ACJ session.
- Aim 3: Explore the feasibility of computing ranks and extracting meaningful portfolio statistics from a subset of ACJ data.
How was the 'Steady State' approach modelled and tested in this study?
Four ACJ sessions were conducted with 13 teachers judging 35 portfolios. Session C, involving all portfolios, served as the "true" rank (analogous to a national assessment). Session B acted as the 'Ruler' (fixed rank). Three models (D1, D2, D3) were used to merge the rank from Session A into Session B by strategically selecting portfolios from Session A to be judged against those in Session B.
What do the Scale Separation Reliability (SSR) values indicate about the models' performance?
SSR measures the reliability of the ranking process. While Model D1 couldn't be assessed due to using only one portfolio, Model D2 yielded an SSR of 0.589, indicating moderate reliability. Surprisingly, Model D3 resulted in a negative SSR (-0.905), demanding further investigation before drawing conclusions.
Which model performed best in merging the ranks and why?
Models D1 and D3 demonstrated similar, strong correlations with the "true" rank, outperforming Model D2. The weaker performance of Model D2 is likely attributed to the higher uncertainty associated with using extreme-ranking portfolios (best and worst), leading to error propagation during rank merging.
What are the practical implications of successfully merging ACJ ranks?
Combining ranks can foster deeper discussions on standard setting and maintenance among stakeholders. Teachers gain insights into exemplar work and associated standards, improving assessment literacy. This also enables the comparison of local and national standards, informing teaching and learning practices.
What are the next steps for refining this 'Steady State' ACJ approach?
Future research will focus on:
- Refining the model for specific assessment scenarios.
- Testing scalability for regional and national assessments.
- Encouraging teacher engagement and adoption of ACJ methodology.
- Developing protocols and technologies to aggregate local assessments for building and maintaining national standards.
References
Buckley, J., Seery, N., & Kimbell, R. (2023). Modelling approaches to combining and comparing independent adaptive comparative judgement ranks. The 40th International Pupils’ Attitudes Towards Technology Conference Proceedings 2023, 1(October).